The Ethical Decision-Making Challenge of AI: Striving for Responsible and Just Algorithms
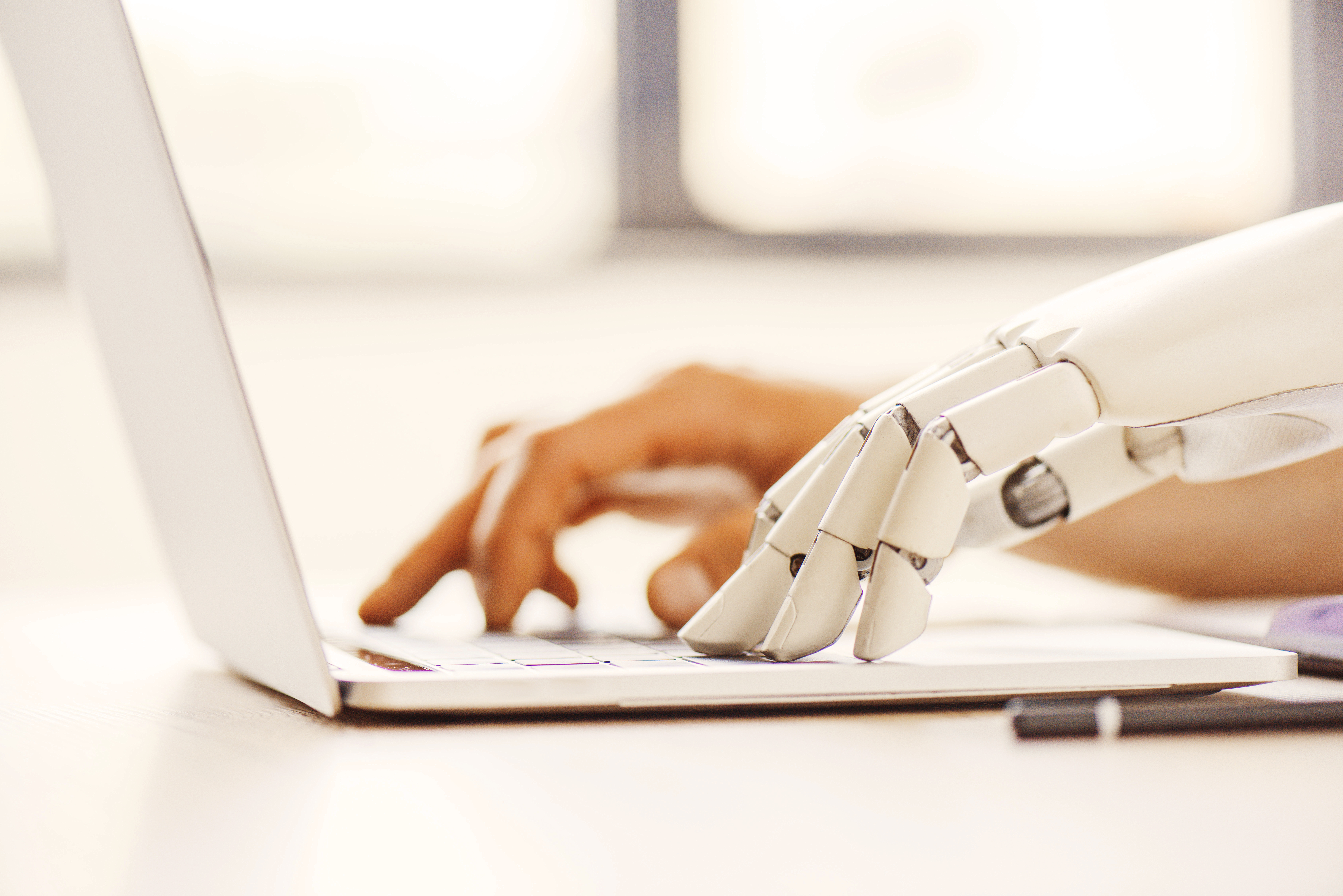
Artificial Intelligence (AI) faces several challenges that need to be addressed to ensure its responsible and ethical development. Here are some key challenges associated with AI:
- Data Bias: AI systems heavily rely on data for training and decision-making. If the training data is biased or contains discriminatory patterns, the AI models can perpetuate those biases, leading to unfair outcomes and discrimination. Addressing data bias requires careful data collection, preprocessing, and ongoing monitoring of AI systems.
- Transparency and Explainability: Many AI algorithms, such as deep learning neural networks, are considered black boxes because they make decisions without providing clear explanations. This lack of transparency can be problematic, especially in high-stakes applications such as healthcare or legal systems, where users need to understand how decisions are made. Developing methods to interpret and explain AI's decision-making processes is crucial for building trust and accountability.
- Ethical Decision-making: AI systems often make decisions that have ethical implications, such as in autonomous vehicles or facial recognition systems. Determining the ethical frameworks and values that guide AI decision-making is a complex challenge. It involves defining and embedding ethical principles into AI algorithms, considering cultural and societal variations, and ensuring AI aligns with human values.
- Security and Privacy: AI systems are susceptible to attacks and can be exploited by malicious actors. Adversarial attacks, where subtle modifications to input data can deceive AI models, pose a significant concern. Additionally, AI's ability to process vast amounts of personal data raises privacy concerns. Protecting AI systems from attacks and ensuring the privacy of individuals' data are ongoing challenges.
- Job Displacement and Workforce Transformation: AI and automation have the potential to disrupt job markets and lead to significant changes in the workforce. While AI can create new opportunities and improve efficiency, it can also replace certain job roles. Preparing the workforce for these changes, providing reskilling opportunities, and addressing the socio-economic impacts of job displacement are crucial challenges.
- Regulation and Policy: Developing appropriate regulations and policies to govern AI technology is a complex task. Balancing innovation and societal well-being requires addressing issues such as AI safety, accountability, liability, and fairness. Governments and organizations are working on creating frameworks that promote responsible AI development and deployment.
- Algorithmic Bias and Fairness: AI systems can exhibit biases, both unintentional and systemic, that result in discriminatory outcomes. These biases can be derived from biased training data, biased design choices, or inherent biases in the algorithms themselves. Ensuring fairness in AI systems and mitigating biases is an ongoing challenge that requires careful scrutiny and evaluation of AI models.
- Limited Generalization and Contextual Understanding: AI models often struggle to generalize their knowledge and understanding from one domain to another or adapt to new and unfamiliar situations. Achieving contextual understanding, common sense reasoning, and robust generalization across various tasks and domains remains a significant challenge in AI research.
Addressing these challenges requires collaborative efforts from researchers, policymakers, and the industry to ensure the development and deployment of AI technologies that are beneficial, fair, and aligned with human values.